A summary of Anesthesiology Grand Rounds from 2 August 2021
On August 2, 2021, I presented at Grand Rounds for our department (Anesthesiology and Perioperative Medicine). For those who missed it (and those outside the department), I’ve prepared a text summary of the presentation.
The first quarter of the presentation focused on the distinction between work that is purely in the realm of statistics compared to projects that require a data science approach. Statistics work is typically focused on detecting relationships in data and quantifying the significance of those relationships. In purely statistical work, hypotheses typically come first. In data science projects, often the reverse is true. Hypotheses are often the outcome of these projects. Data Science projects are often focused on making a prediction. After a model is developeds, hypotheses about why the model is good at making predictions (if it is) are formed and can spur future research. Data science tends to be hypothesis-generating rather than hypothesis-testing. For an expand discussion see my previous post on the topic of statistics vs machine learning specifically.
As an example of a Data Science project, I repeated a 5-minute presentation I gave at SOCCA’s (Society of Critical Care Anesthesiologists) annual meeting in May of this year. The talk itself is available on demand for those who attended SOCCA’s annual meeting in 2021. In this project, we explored whether machine learning techniques can predict incidence of allogenic blood transfusion products and identify important risk factors. This was a data science project because it started with a question (as opposed to a hypothesis), addressed a extant data set that might contain the answer, and assessed connections between patient outcomes and features after models making predictions were trained.
I summed up this section of the talk a rule of thumb: “Statistics is a hypothesis in search of data; whereas, data science is data or predictions in search of a hypothesis.”
The next section focused on business understanding as the key ingredient in data science projects. Knowing the question we’re trying to answer and the business purpose or significance of it is the primary focus of data science projects. Building models (be they statistical or machine learning) is one small part of data science work. This is best visualized using the CRoss Industry Standard Process for Data Mining (CRISP-DM) project cycle (below).
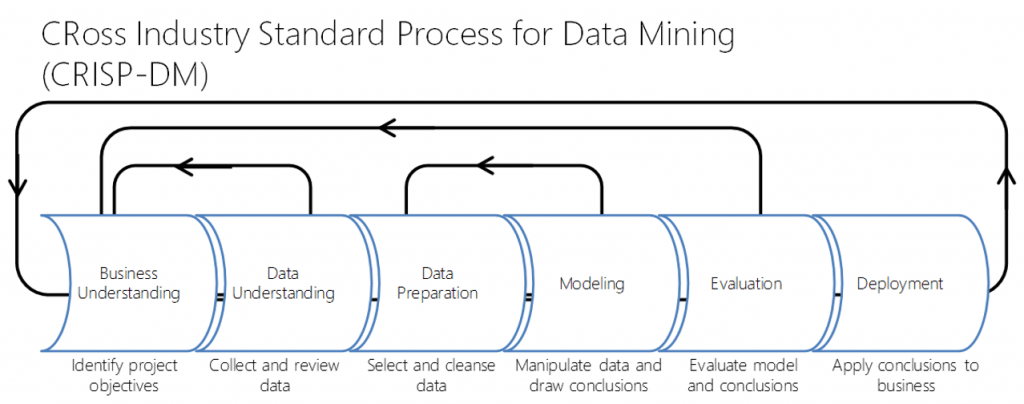
I then gave some concrete examples of outcomes of data science projects. For example, a project might result in a preoperative warning and risk assessment system (example below).
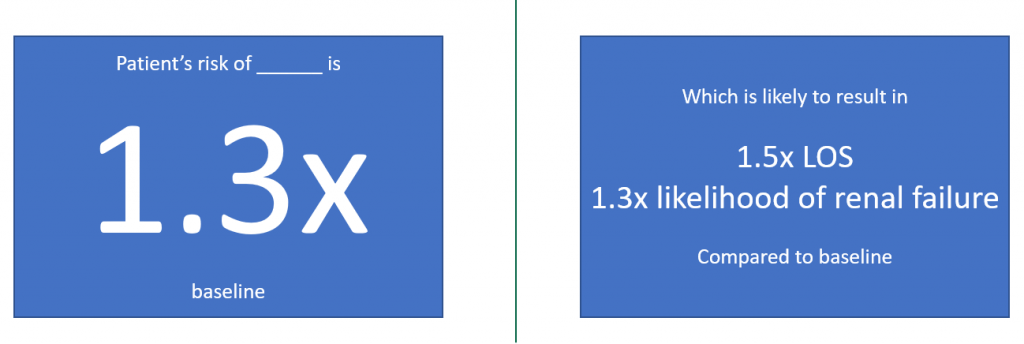
Next, I discussed our high-resolution, real-time data capture and analysis platform Sickbay, which I’ve posted about before. We recently submitted our first manuscript with data and analysis from the Sickbay system.
I reviewed the collaborations Data Science has brought to the department over the last year and closed the presentation with guidance on getting connected (slide below).
