
The problem of liver fibrosis is widespread, and the need for objective tools for fibrosis detection and evaluation is obvious. In this research project, we are trying to provide tools for robust liver fibrosis staging, based on diffusion MRI image analysis.
The current practice of fibrosis assessment, which is based on painful liver biopsy, might be dangerous. Moreover, the decision of the histopathologist based on a biopsy is subjective, and depends on the sample, because the fibrosis level varies along the liver. No objective standard has been developed yet for histological fibrosis assessment.
Magnetic resonance volume data has much lower resolution than histological image data, but it includes the entire liver volume. Also, MRI is non-invasive and not painful, thus, it is preferred as a diagnostic tool. Previously it has been hypothesized that the average brightness of Apparent Diffusion Coefficient (ADC) in diffusion MRI correlates with the fibrosis stage.
In our research, we have tested different ADC image texture features and have found features (e.g., standard deviation of color distribution) that correlate with histological results much better than average brightness. We have developed an algorithm for automatic hepatic MRI segmentation, and algorithm for automatic liver staging, based on optimal feature grouping.
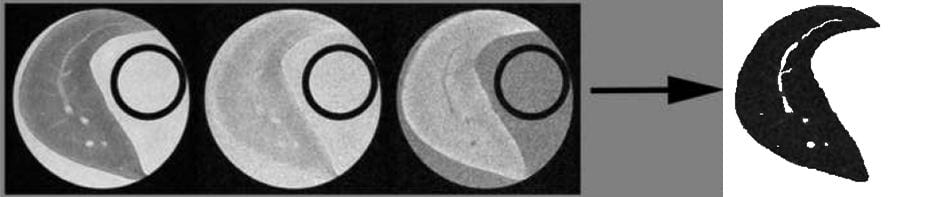
- B. Barry, K. Buch, J. Soto, H. Jara, A. Nakhmani, S. Anderson, “Quantifying Liver Fibrosis Through the Application of Texture Analysis to Diffusion Weighted Imaging.” Magnetic Resonance Imaging Journal, Volume 32, Issue 1, pp. 84–90, January 2014.
Published by